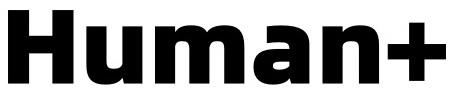
Touch-and-Heal: Data-driven Affective Computing in Tactile Interaction with Robotic Dog
Shihui Guo*, Lishuang Zhan*, Yancheng Cao, Chen Zheng, Guyue Zhou, Jiangtao Gong†
UbiComp/IMWUT 2023
Abstract
Affective touch plays an important role in human-robot interaction. However, it is challenging for robots to perceive various natural human tactile gestures accurately, and feedback human intentions properly. In this paper, we propose a data-driven affective computing system based on a biomimetic quadruped robot with large-format, high-density flexible pressure sensors, which can mimic the natural tactile interaction between humans and pet dogs. We collect 208-minute videos from 26 participates and construct a dataset of 1212 human gestures-dog actions interaction sequences. The dataset is manually annotated with an 81-tactile-gesture vocabulary and a 44-corresponding-dog-reaction vocabulary, which are constructed through literature, questionnaire, and video observation. Then, we propose a deep learning algorithm pipeline with a gesture classification algorithm based on ResNet and an action prediction algorithm based on Transformer, which achieve the classification accuracy of 99.1% and the 1-gram BLEU score of 0.87 respectively. Finally, we conduct a field study to evaluate the emotion regulation effects through tactile affective interaction, and compare it with voice interaction. The results show that our system with tactile interaction plays a significant role in alleviating user anxiety, stimulating user excitement and improving the acceptability of robotic dogs.
BibTex