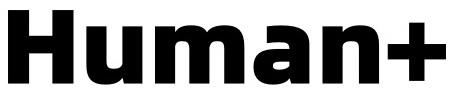
Loose Inertial Poser: Motion Capture with IMU-attached Loose-Wear Jacket
Chengxu Zuo, Yiming Wang, Lishuang Zhan, Shihui Guo*, Xinyu Yi, Feng Xu, Yipeng Qin
Computer Vision and Pattern Recognition 2024
Abstract
Existing wearable motion capture methods typically demand tight on-body fixation (often using straps) for reliable sensing, limiting their application in everyday life. In this paper, we introduce Loose Inertial Poser, a novel motion capture solution with high wearing comfortableness, by integrating four Inertial Measurement Units (IMUs) into a loose-wear jacket. Specifically, we address the challenge of scarce loose-wear IMU training data by proposing a Secondary Motion AutoEncoder (SeMo-AE) that learns to model and synthesize the effects of secondary motion between the skin and loose clothing on IMU data.
Our SeMo-AE consists of two novel techniques: i) noise-guided latent space learning, which reverses the process of noise modeling to instead model the latent space, thus enabling easy extrapolation of secondary motion effects; ii) a temporal coherence strategy that models the continuity of secondary motions across successive frames. SeMo-AE is then leveraged to generate a diverse synthetic dataset of loose-wear IMU data to augment training for the pose estimation network and significantly improve its accuracy. For validation, we collected a dataset with various subjects and 2 wearing styles (zipped and unzipped). Experimental results demonstrate that our approach maintains high-quality real-time posture estimation even in loose-wear scenarios.
BibTex
@inproceedings{zuo2024loose,
title={Loose inertial poser: Motion capture with IMU-attached loose-wear jacket},
author={Zuo, Chengxu and Wang, Yiming and Zhan, Lishuang and Guo, Shihui and Yi, Xinyu and Xu, Feng and Qin, Yipeng},
booktitle = {Computer Vision and Pattern Recognition},
year={2024}
}